CAMBRIDGE, Mass. — Artificial intelligence (AI) has identified a new class of compounds capable of eliminating the hospital superbug MRSA, according to researchers at the Massachusetts Institute of Technology. They utilized an AI model to identify compounds that combat the drug-resistant bacterium, notorious for causing up to 120,000 global deaths annually. Concerningly, this bacterium often spreads throughout hospitals.
The scientists employed a robotic intelligence model, gaining insights into the data types used by the deep-learning model for predicting antibiotic effectiveness.
Remarkably, these compounds exhibit minimal toxicity to human cells, deeming them excellent drug candidates. This study, part of MIT’s Antibiotics-AI Project, aims to discover new antibiotic classes against seven deadly bacteria types over seven years.
Methicillin-resistant Staphylococcus aureus (MRSA), infecting over 80,000 people yearly in the United States, can cause skin infections or pneumonia. Severe cases may lead to fatal bloodstream infections known as sepsis.
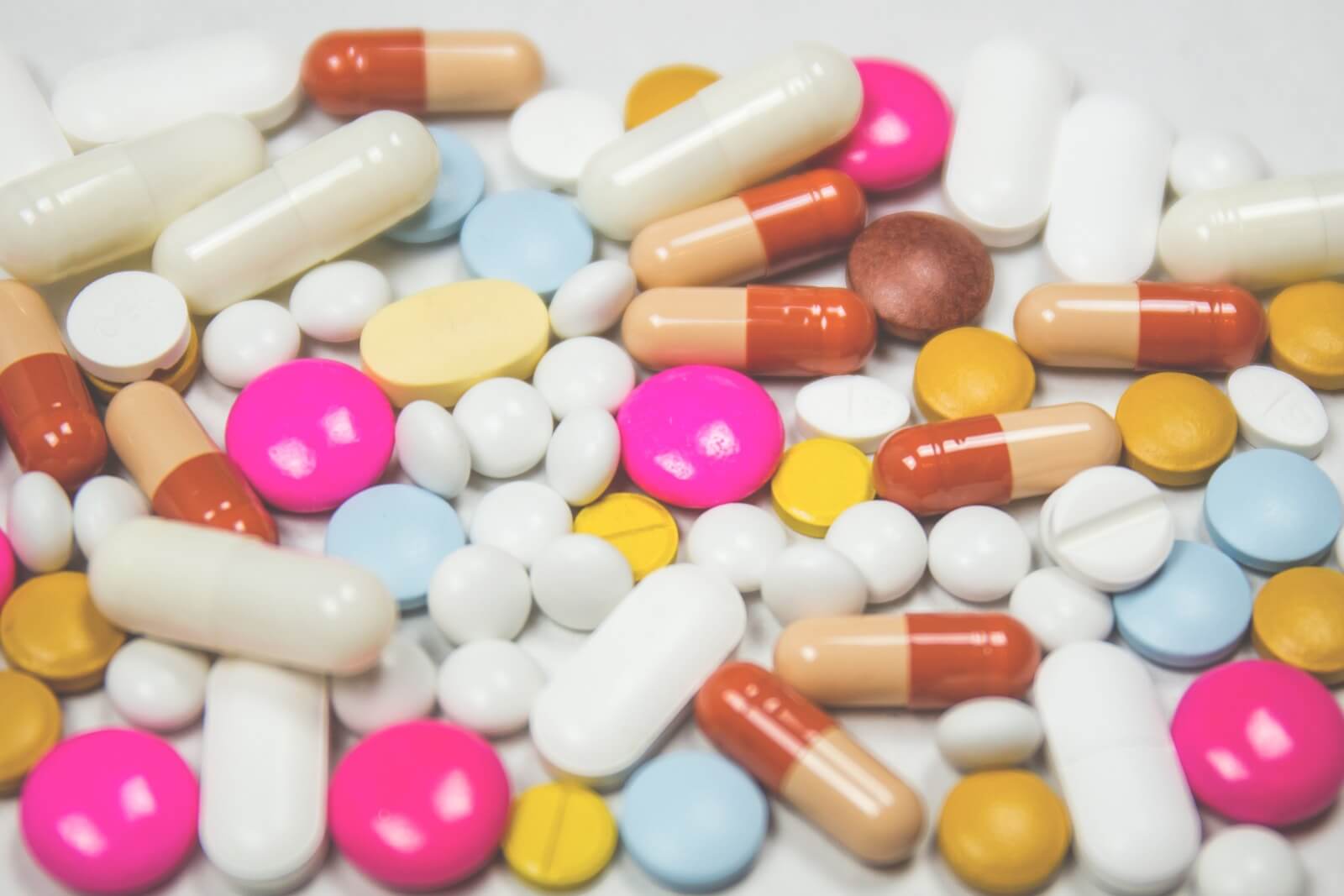
MIT researchers have recently adopted deep learning, a type of AI mimicking human learning processes, to discover new antibiotics. Their efforts have identified potential drugs against Acinetobacter baumannii and other drug-resistant bacteria, found commonly in hospitals.
These potential drugs were pinpointed using deep learning models that recognize chemical structures linked to antimicrobial activity. These models analyze millions of compounds, predicting those with potent antimicrobial properties. However, these models are “black boxes,” obscuring the basis of their predictions.
“What we set out to do in this study was to open the black box,” says Dr. Felix Wong from the Broad Institute of MIT and Harvard, in a media release. “These models consist of very large numbers of calculations that mimic neural connections, and no one really knows what’s going on underneath the hood.”
The team trained a deep learning model with extensive datasets, including tests on approximately 39,000 compounds for MRSA antibiotic activity. This data, alongside chemical structure information, was fed into the model.
They adapted the Monte Carlo tree search algorithm, previously used in other deep learning models, to decipher the model’s prediction process. Additionally, three more models were trained to assess compound toxicity to human cells. This approach led to the discovery of compounds with high antimicrobial activity and low human toxicity.
“Our substantially augmented deep learning approach allowed us to predict this new structural class of antibiotics and enabled the finding that it is not toxic against human cells,” Dr. Wong adds.
Screening around 12 million compounds using AI, the MIT team identified promising candidates from five different classes against MRSA. Out of 280 tested compounds, two showed significant potential in laboratory and mouse model tests, reducing MRSA populations by a factor of 10.
These compounds disrupt bacterial cell membrane electrochemical gradients, selectively targeting bacterial cells without substantially harming human cells.
“The insight here was that we could see what was being learned by the models to make their predictions that certain molecules would make for good antibiotics,” Professor James Collins of MIT highlights. “Our work provides a framework that is time-efficient, resource-efficient, and mechanistically insightful, from a chemical-structure standpoint, in ways that we haven’t had to date.”
The study is published in the journal Nature.
You might also be interested in:Â
- Antibiotics can turn your gut into a breeding ground for drug-resistant superbugs
- Hospital floors are hotspots for bacteria, allowing quick spread to surfaces and people
- Antibiotic-resistant bacteria contaminates an entire hospital ICU
South West News Service writer James Gamble contributed to this report.
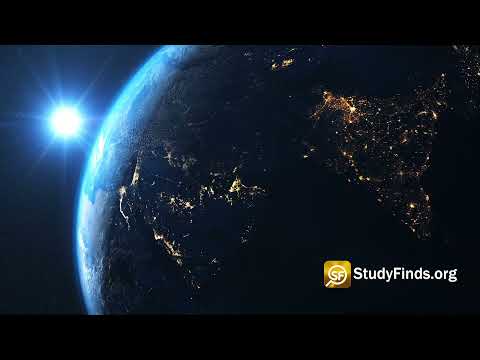